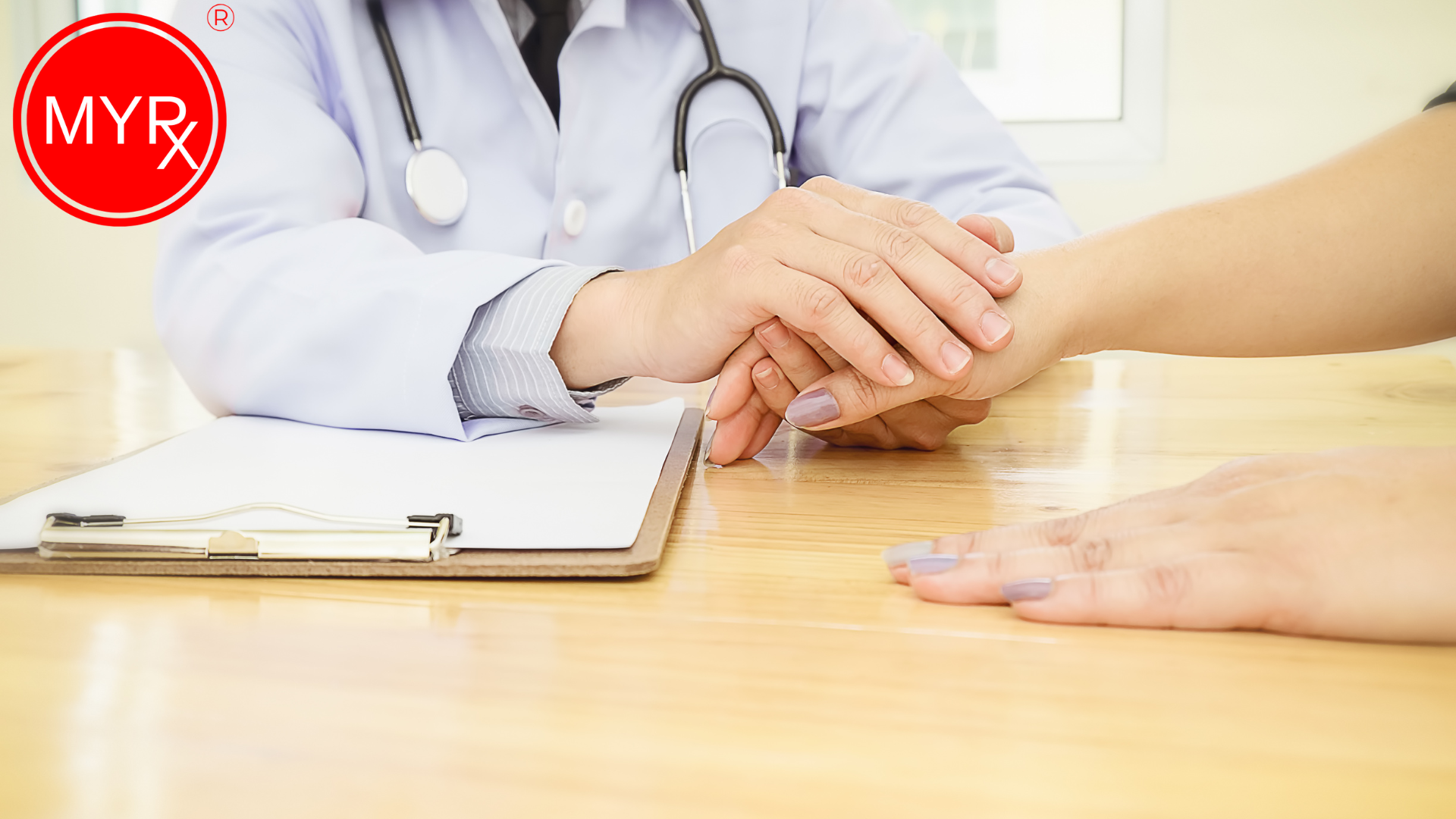
The Role of Artificial Intelligence in Drug Discovery: Accelerating Drug Development and Innovation
Introduction
The process of drug discovery and development is complex, time-consuming, and costly. However, with the advent of Artificial Intelligence (AI), the landscape of drug discovery is rapidly evolving. AI is revolutionizing various stages of the drug development pipeline, from target identification to clinical trials, by leveraging its capabilities in data analysis, pattern recognition, and predictive modeling. In this article, we will explore the role of AI in drug discovery and how it is accelerating the development of new drugs and fostering innovation in the pharmaceutical industry.
Target Identification and Validation:
AI algorithms can analyze vast amounts of biological data, including genomics, proteomics, and electronic health records, to identify potential drug targets. By mining these datasets and identifying patterns, AI can prioritize promising targets with a higher probability of success. This targeted approach streamlines the initial stages of drug discovery, enabling researchers to focus their efforts on the most promising candidates.
Drug Design and Optimization:
AI techniques, such as machine learning and deep learning, can aid in the design and optimization of drug molecules. AI algorithms can analyze the structure-activity relationships of known compounds, predict the effectiveness of new compounds, and optimize their properties for improved drug efficacy and safety. This computational modeling approach reduces the reliance on time-consuming and expensive experimental methods, accelerating the drug design process.
Virtual Screening and Drug Repurposing:
AI enables virtual screening, where large databases of molecules are screened computationally to identify potential drug candidates. AI algorithms can predict the likelihood of a molecule binding to a target protein, allowing researchers to prioritize the most promising compounds for further investigation. Additionally, AI can facilitate drug repurposing by identifying existing drugs that may have therapeutic effects for different diseases, potentially reducing the time and cost required for clinical development.
Predictive Toxicity Assessment:
AI algorithms can assess the potential toxicity of drug candidates, minimizing the risk of adverse effects during clinical trials. By analyzing large datasets of known toxic compounds and their associated features, AI can predict the toxicity profiles of new compounds. This predictive toxicity assessment helps researchers make informed decisions about which compounds to advance in the drug development process, improving safety and reducing late-stage failures.
Clinical Trial Optimization:
AI can optimize the design and execution of clinical trials, improving efficiency and increasing the chances of success. AI algorithms can analyze patient data, identify suitable trial populations, and optimize trial protocols. By incorporating real-time data from ongoing trials, AI can also enable adaptive trial designs, where protocols can be adjusted based on emerging results, enhancing the probability of successful outcomes.
Personalized Medicine and Treatment Response Prediction:
AI has the potential to advance personalized medicine by analyzing patient data and predicting individual treatment responses. By integrating genomic information, clinical data, and other relevant factors, AI algorithms can help identify patient subgroups that are likely to respond positively to specific treatments. This enables more targeted and effective therapies, reducing trial and error in treatment selection.
Drug Safety Monitoring and Pharmacovigilance:
AI can contribute to post-marketing surveillance and pharmacovigilance by analyzing real-world data to identify potential safety concerns associated with approved drugs. By monitoring adverse events, drug interactions, and other factors, AI algorithms can help identify and mitigate risks, improving patient safety.
Conclusion
Artificial Intelligence is transforming the field of drug discovery by accelerating the development of new drugs, fostering innovation, and improving patient outcomes. From target identification to clinical trial optimization and personalized medicine, AI offers powerful tools for analyzing complex biological data, predicting drug efficacy and toxicity, and optimizing various stages of the drug development pipeline. As AI continues to advance and integrate with pharmaceutical research, we can expect a more efficient, cost-effective, and targeted approach to drug discovery, ultimately leading to the development of novel therapies for a wide range of diseases.