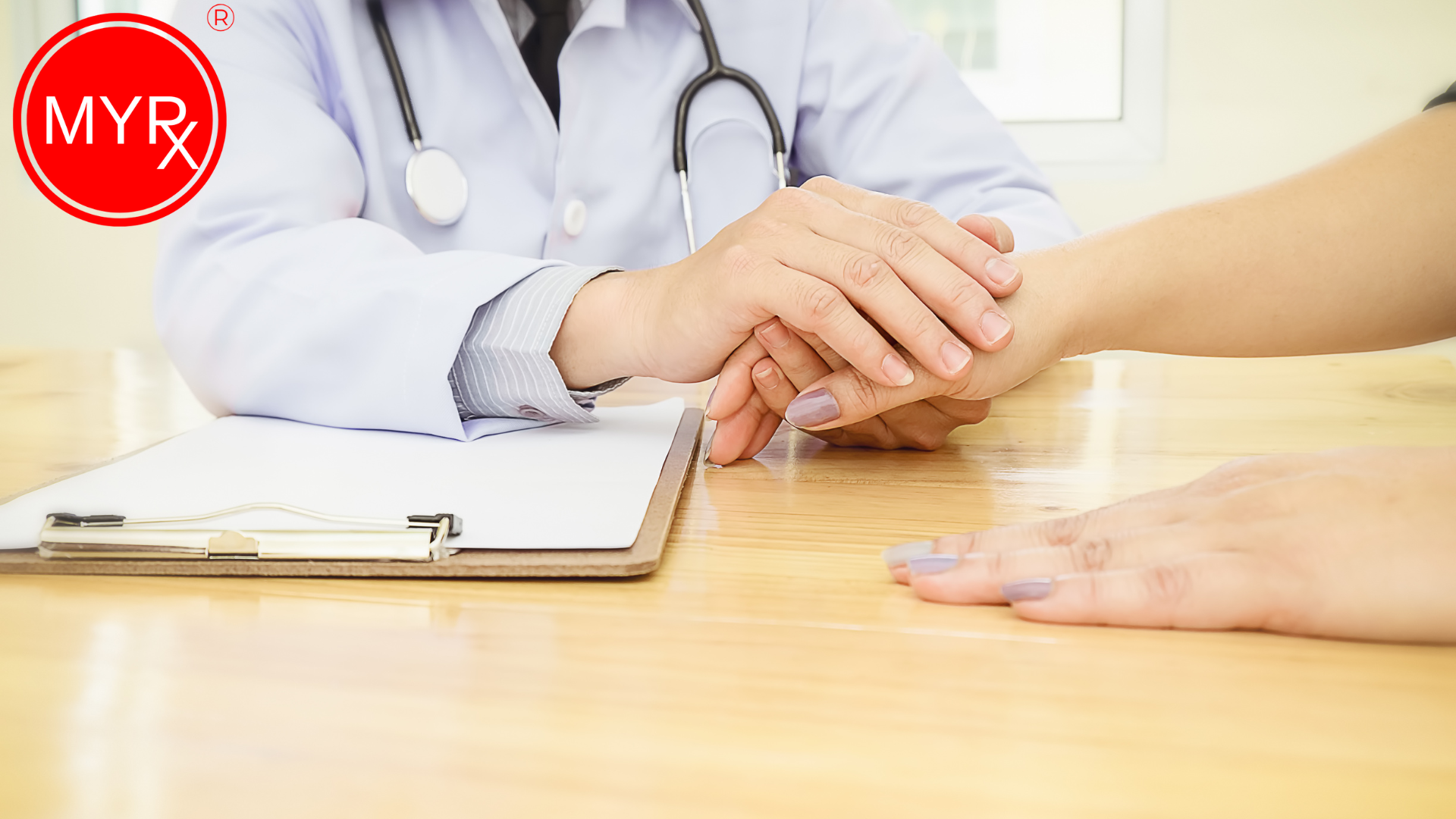
Using AI and Machine Learning for Predictive Analytics in Healthcare
Introduction
Artificial Intelligence (AI) and Machine Learning (ML) are revolutionizing healthcare by enabling predictive analytics, a powerful tool for improving patient outcomes, optimizing resource allocation, and driving operational efficiency. By leveraging AI and ML algorithms, healthcare providers can analyze large datasets, identify patterns, and make accurate predictions about diseases, patient risks, and treatment outcomes. In this article, we will explore the applications and benefits of using AI and ML for predictive analytics in healthcare.
Early Disease Detection and Prevention:
AI and ML algorithms can analyze vast amounts of patient data, including electronic health records, diagnostic test results, and genetic information, to identify early signs of diseases. By detecting patterns and risk factors, predictive analytics can enable early intervention and preventive measures, leading to improved patient outcomes and reduced healthcare costs.
Personalized Treatment Plans:
Predictive analytics powered by AI and ML can help healthcare providers develop personalized treatment plans for patients. By considering individual patient characteristics, medical history, and predictive models, clinicians can tailor treatments to maximize effectiveness and minimize adverse effects. This patient-centric approach enhances treatment outcomes and patient satisfaction.
Resource Optimization and Operational Efficiency:
AI and ML algorithms can analyze historical data and current patient trends to predict future resource demands, such as hospital bed occupancy, staffing requirements, and equipment utilization. This enables healthcare organizations to optimize resource allocation, streamline operations, and improve cost-effectiveness without compromising patient care.
Risk Stratification and Patient Management:
Predictive analytics can assist in risk stratification by identifying patients at high risk of developing complications, readmissions, or adverse events. By flagging at-risk individuals, healthcare providers can proactively intervene, implement targeted interventions, and allocate resources accordingly, improving patient management and reducing healthcare utilization.
Medication Adherence and Disease Management:
AI and ML algorithms can predict medication adherence rates based on patient data, socioeconomic factors, and behavioral patterns. By identifying patients at risk of non-compliance, healthcare providers can intervene with tailored interventions, reminders, or educational programs, improving medication adherence and disease management outcomes.
Clinical Decision Support Systems:
Integrating predictive analytics into clinical decision support systems empowers healthcare providers with real-time, data-driven insights at the point of care. AI and ML algorithms can provide evidence-based recommendations, risk scores, and treatment guidelines, assisting clinicians in making informed decisions and improving diagnostic accuracy.
Clinical Trials and Drug Development:
Predictive analytics can enhance the efficiency of clinical trials and drug development processes. AI and ML algorithms can identify patient populations most likely to respond to a particular treatment, enabling targeted recruitment and optimizing trial outcomes. This helps accelerate the development and approval of new therapies, benefiting patients and advancing medical science.
Conclusion
AI and ML-driven predictive analytics have the potential to revolutionize healthcare by enabling early disease detection, personalized treatment plans, and optimized resource allocation. With the ability to analyze vast amounts of data, identify patterns, and make accurate predictions, AI and ML algorithms empower healthcare providers to deliver proactive, patient-centric care and improve overall healthcare outcomes. As technology continues to advance, the integration of predictive analytics in healthcare is expected to become increasingly valuable in enhancing clinical decision-making, patient management, and operational efficiency.